Citizen science: become part of a real-time Global Landslide Detector
BGS and partners are trialling a new social media tool that detects worldwide landslides in real time.
29/08/2022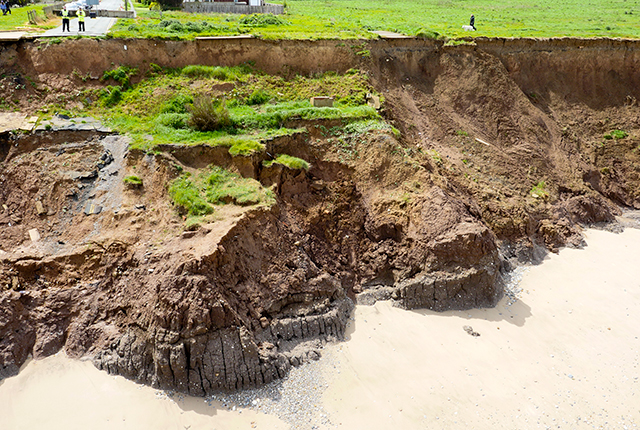
Social media is a valuable tool. Society is now sharing a constant stream of real-time information across a wide variety of digital platforms. Alongside all the pictures of pets, family members and delicious dinners, social media channels are also used when disaster strikes. In this respect, social media has the power to harness important data that can have a huge impact for the global good. Take, for instance, the ability to learn about natural disasters across the world, as they unfold in real time.
BGS has been working with earthquake and social media specialists at the European-Mediterranean Seismological Centre and computer scientists at the Qatar Computing Research Institute to build the Global Landslide Detector, a tool that uses machine learning to recognise landslides in photographs shared on social media.
When a landslide happens, the damage caused is usually unknown beyond the locality until news reporters can attend the scene or once satellites have been able to collect images, and their responding communities have processed the data. This is called ‘data latency’ and can take some time – at best a few hours; at worst, several days.
We realised we could help improve this situation by speeding up the timescale in which landslide data becomes available on a global scale.
Why is social media useful for landslides research?
Social media information is imperfect; there is an awful lot of content being produced very quickly and it is constantly being added to. While this can seem a little overwhelming, if you have a way of untangling valuable updates from the social noise, what you will end up with is data in large quantities, in real time, covering geographical areas much larger than any conventional ground sensors can detect. These ‘social sensors’ allow access to a rich source of human information such as text, videos, photographs, timestamps and locations. This can provide us with disaster information very quickly.
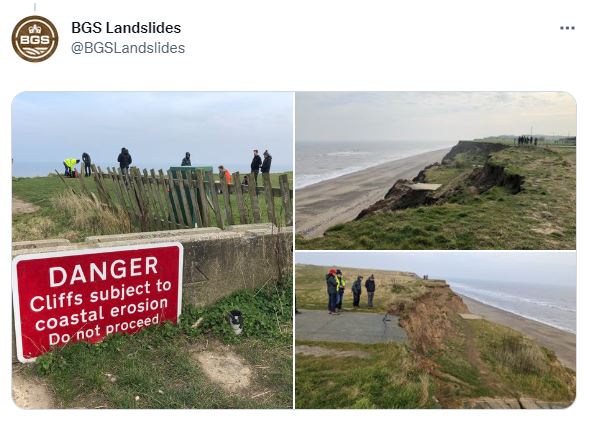
A typical tweet from the @BGSLandslides team. BGS © UKRI.
The Global Landslide Detector is a publicly available web service that extracts, in real time, photographs of landslides published on social media. This is something we hope will be welcomed by various sectors, including disaster risk reduction managers, first responders and landslide database researchers.
The detector currently monitors Twitter only, although there is scope for expansion in the future. It extracts tweets that contain a photograph in association with particular keywords. The keywords we’ve inputted are currently available in 31 different languages, which means that translation shouldn’t be a barrier to using the tool. Using machine learning, the tool then analyses the photographs automatically, to decide whether they contain a landslide or not. The data from this process is then organised by location and markers are placed on a map.
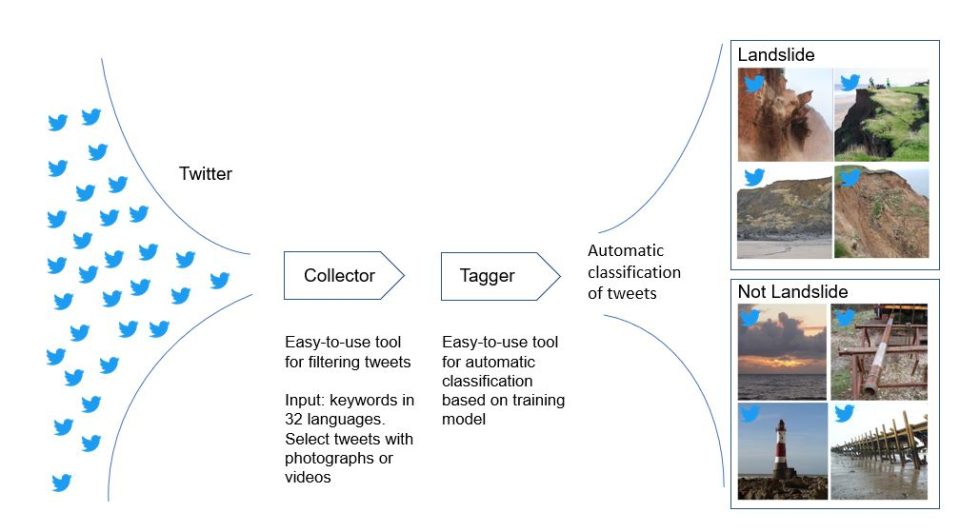
Diagram showing how the Global Landslide Detector extracts relevant information from Twitter and sorts photographs into landslides and not landslides. BGS © UKRI.
How you can help
Currently we are trialling the first version of the tool on the website. We are asking for feedback on several elements from the scientific landslide community, as well as anyone else with an active interest, including:
- refining the landslide keywords used to extract tweets
- expanding and updating keywords in other languages
- general layout
You can find an initial feedback form on the website, which may lead to further discussion, if needed.
Limitations
Before using the tool, it is important to understand some of its limitations.
- The Global Landslides Detector does not replace expert survey; data can come from any social media user
- Each photograph is analysed in isolation
- The tool does not discriminate between landslide ‘types’ but aims to recognise zones of depletion (where the material has come from) and accretion (where it has been deposited); this excludes scenarios where the landslide debris has been removed by coastal or fluvial erosion, or where a landslide has been remediated
- The tool aims to show contemporary, rather than historic, landslides
- There must be a clear representation of a landslide as the major focus of the image
- Where representation is borderline, consideration is given to whether the end user would be concerned by the image being labelled as a landslide, e.g. in a situation where another geomorphological feature such as a retaining wall or a sinkhole might be marked as a landslide
- The methodology is aimed to identify landslide features, but the task is not to discriminate scale, meaning that images labelled as landslides may be very small (less than 1 m and not strictly a landslide)
- Aerial photographs including multiple landslide events are not captured by the tool
- The tool is not intended to be used in isolation during a disaster scenario but could complement existing workflows and provide new data, taking into consideration the limitations described and data biases (e.g. mobile coverage; widespread use of social media; population density)
The Global Landslide Detector website is not under the control of BGS and BGS is not responsible for the website, policies or practices.
Further reading
Pennington, C V L, Bossu, R, Ofli, F, Imran, M, Qazi, U W, Roch, J, and Banks, V. 2022. A near-real-time global landslide incident reporting tool demonstrator using social media and artificial intelligence. International Journal of Disaster Risk Reduction, Vol. 77, 103089. DOI: https://doi.org/10.1016/j.ijdrr.2022.103089
Ofli, F, Imran, M, Qazi, U, Roch, J, Pennington, C V L, Banks, V, and Bossu, R. 2022. Landslide detection in real-time social media image streams. The Thirty-Fourth Annual Conference on Innovative Applications of Artificial Intelligence (IAAI-22), February 24-26, 2022, Vancouver, BC, Canada. Available at: https://arxiv.org/pdf/2110.04080.pdf

Catherine Pennington
Engineering geologist, landslide specialist
Latest blogs
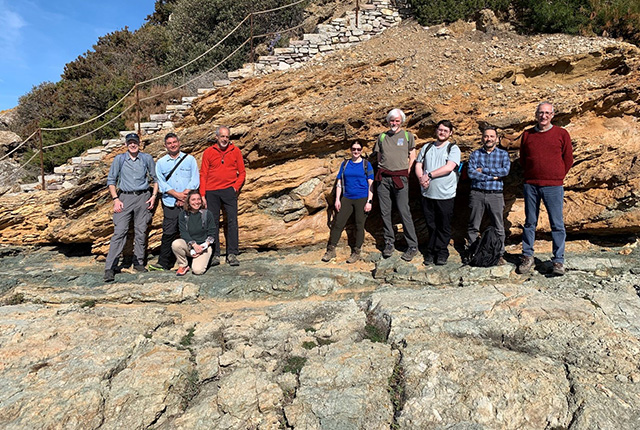
The heat beneath our feet: BGS field visit to Tuscan geothermal systems
05/07/2024
BGS visits the active and fossilised geothermal systems of southern Tuscany, Italy.
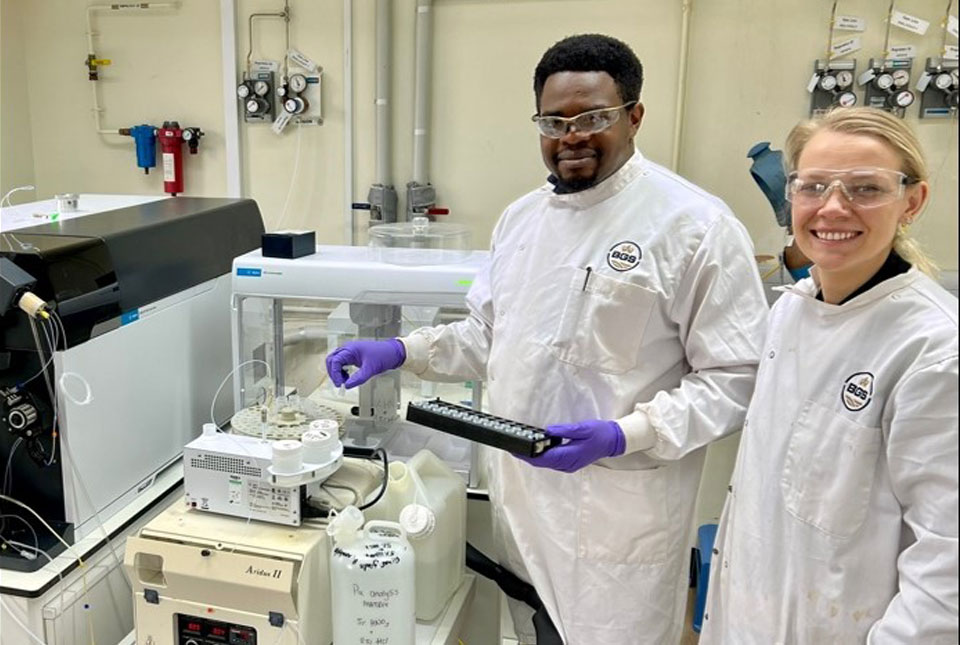
Harnessing global collaboration: UK/Kenya partnership in soil erosion research
31/05/2024
Collaboration between scientists is vital in today’s interconnected world to further scientific progress. In environmental research, issues such as soil erosion demand collaboration on an international scale.
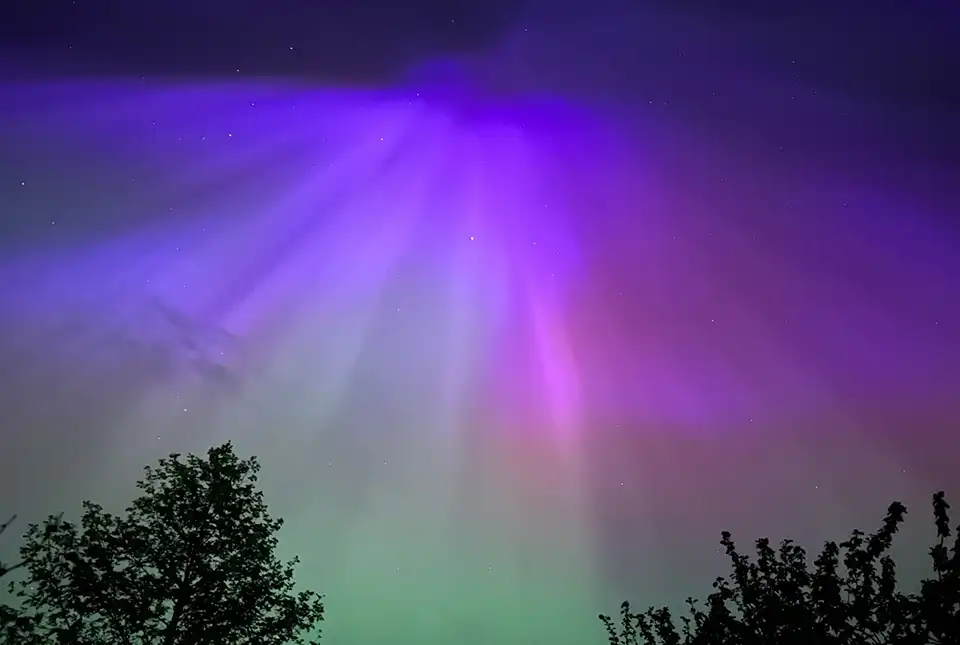
A-roaring display
16/05/2024
The dazzling and colourful aurora borealis, or northern lights, observed by many across the UK last weekend was one of the most extreme and long-lasting geomagnetic storms recorded in the last 155 years.
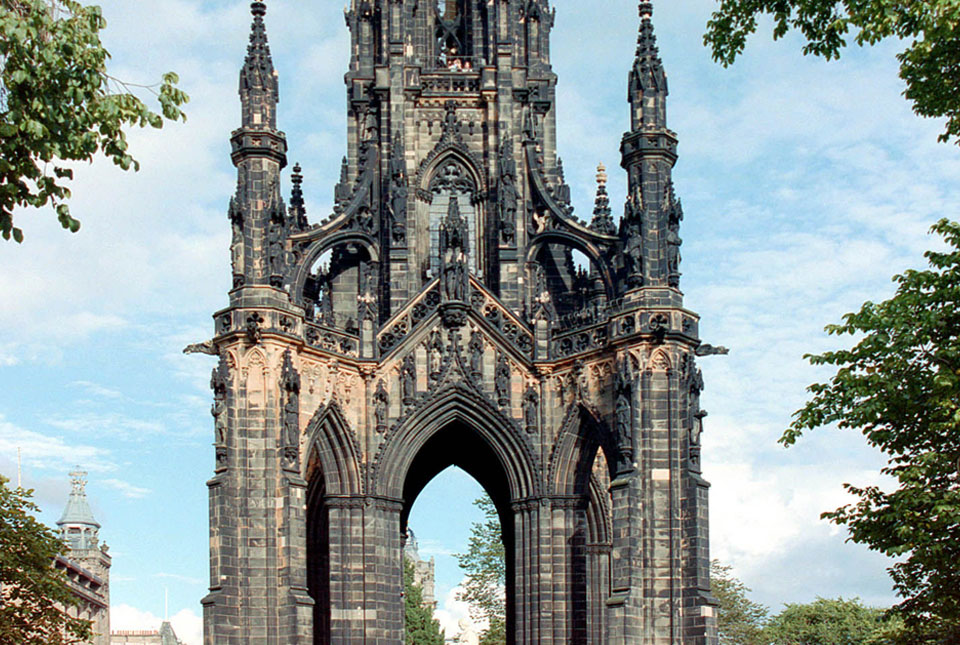
Building stones spotlight: the Sir Walter Scott Memorial 25 years after its conservation
29/04/2024
BGS geologist, Luis Albornoz-Parra, discusses the iconic Edinburgh monument, the building stones used in its construction and the result (so far) of its conservation efforts.
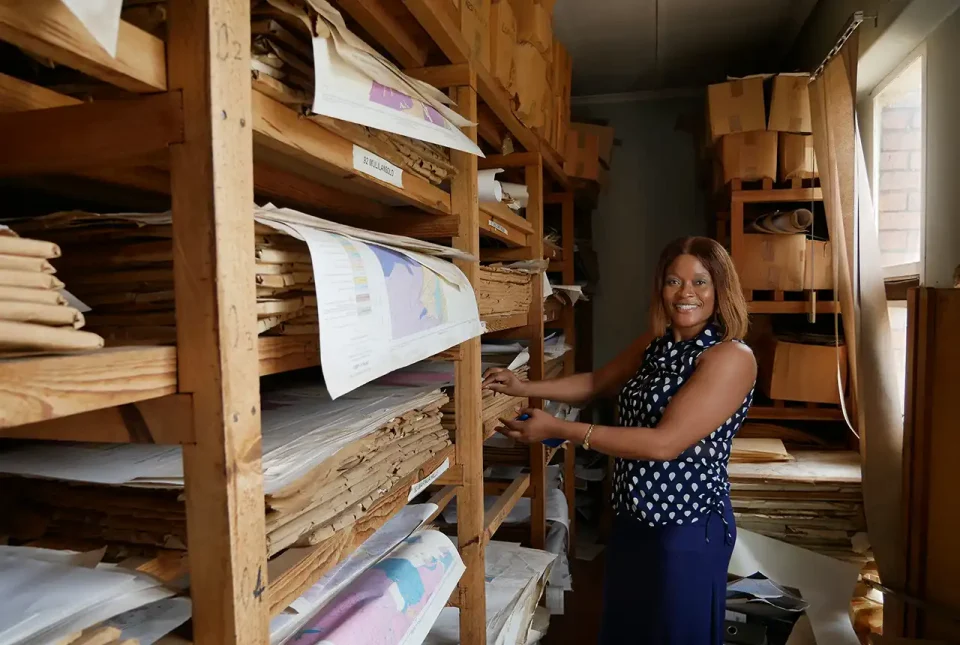
Unlocking key mineral archives at the Zambian Geological Survey Department
23/02/2024
Rachel Talbot recounts a recent visit by BGS Records staff to the Zambian Geological Survey Department, to assist in critical mineral data management.
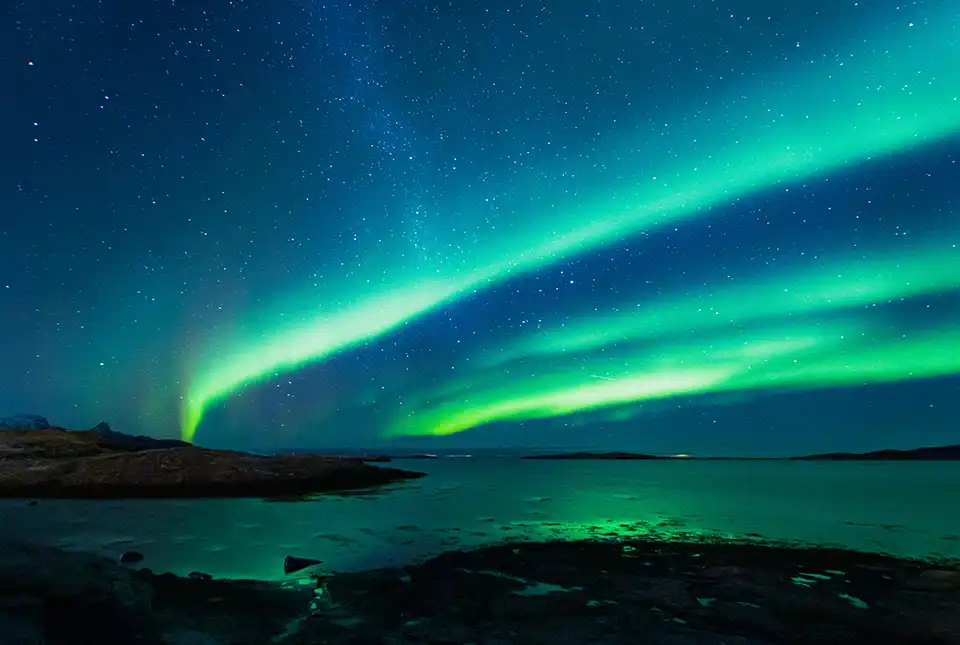
Will 2024 be the Year of the Aurora?
23/02/2024
The Sun’s approximate eleven-year activity cycle is predicted to peak this year, prompting BGS scientists to anticipate that 2024 will be the ‘Year of the Aurora’.
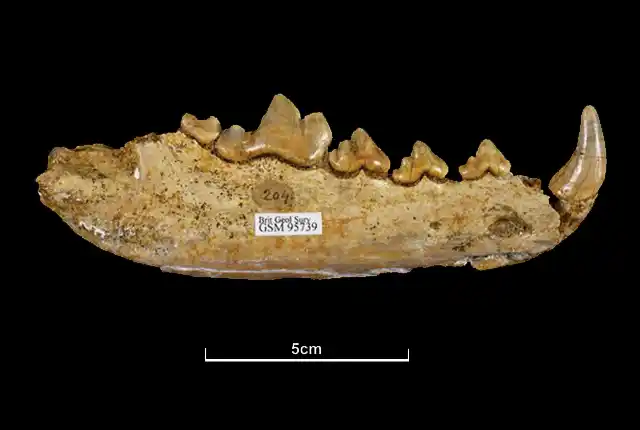
Hungry like a wolf: new insights from old bones housed in the BGS museum collections
18/01/2024
BGS scientists are studying the diets of ancient British wolves and how they adapted to changing environments.
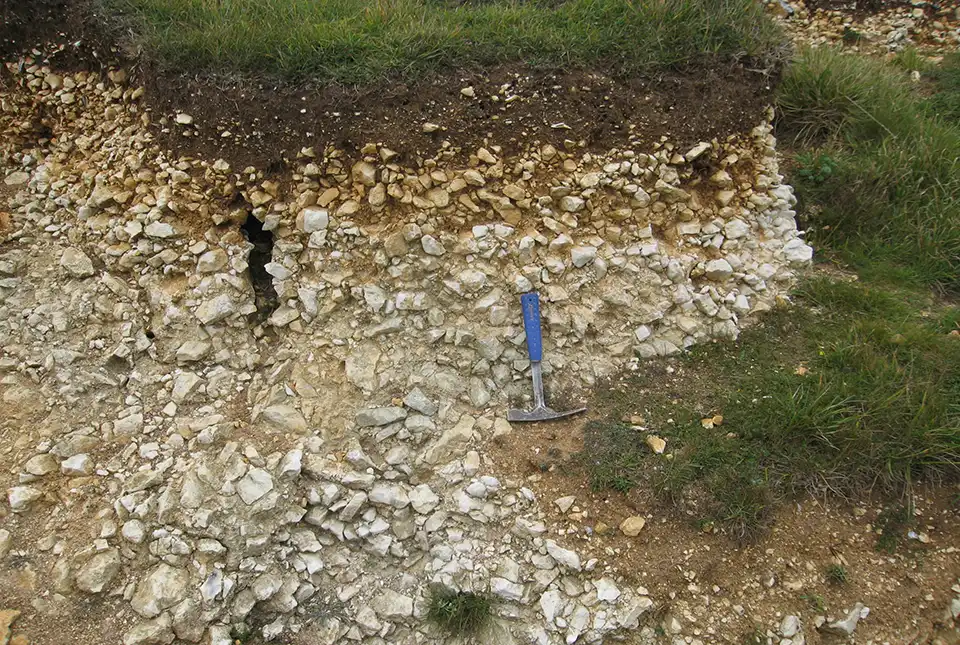
How BGS is helping the farming sector of Great Britain
17/01/2024
New legislation concerning soil management and technology in modern farming has led to an increase in enquiries about BGS’s Soil Parent Material Model.
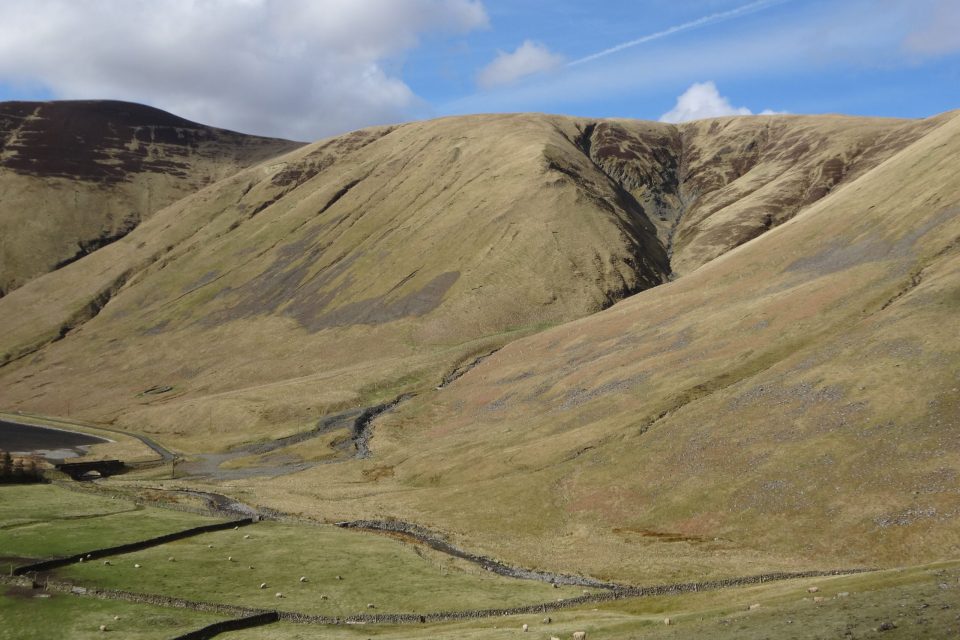
Moving stones: faults, slopes and sediments
12/01/2024
Fractured rock along faults affects sediment movement on slopes with implications for the design of infrastructure.
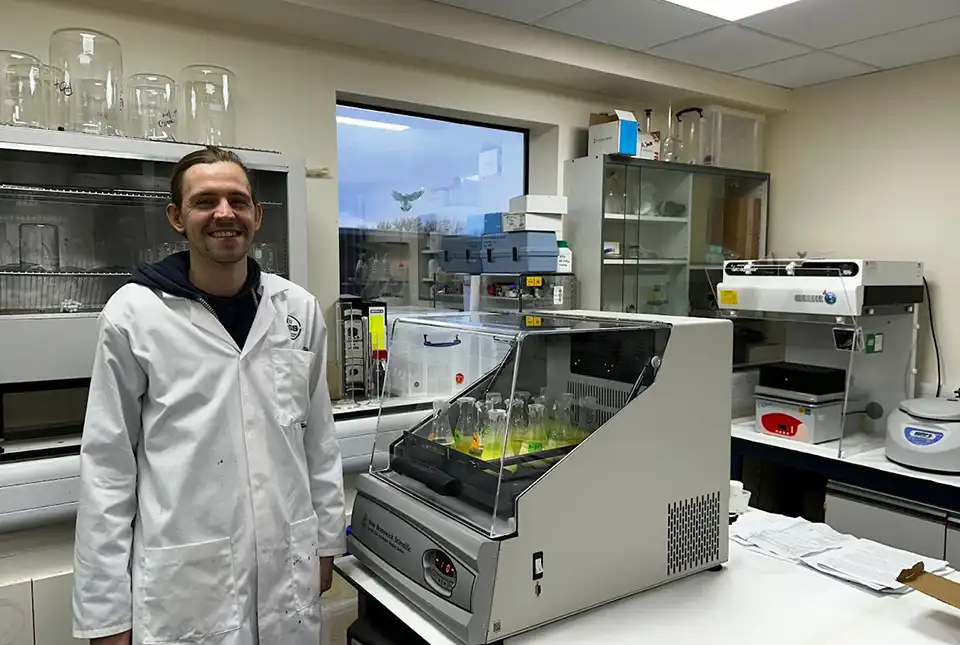
Understanding nutrients in tropical rainforests
11/01/2024
Christopher Bengt talks about carrying out research for his PhD amongst the rainforests and volcanoes of the Philippines.
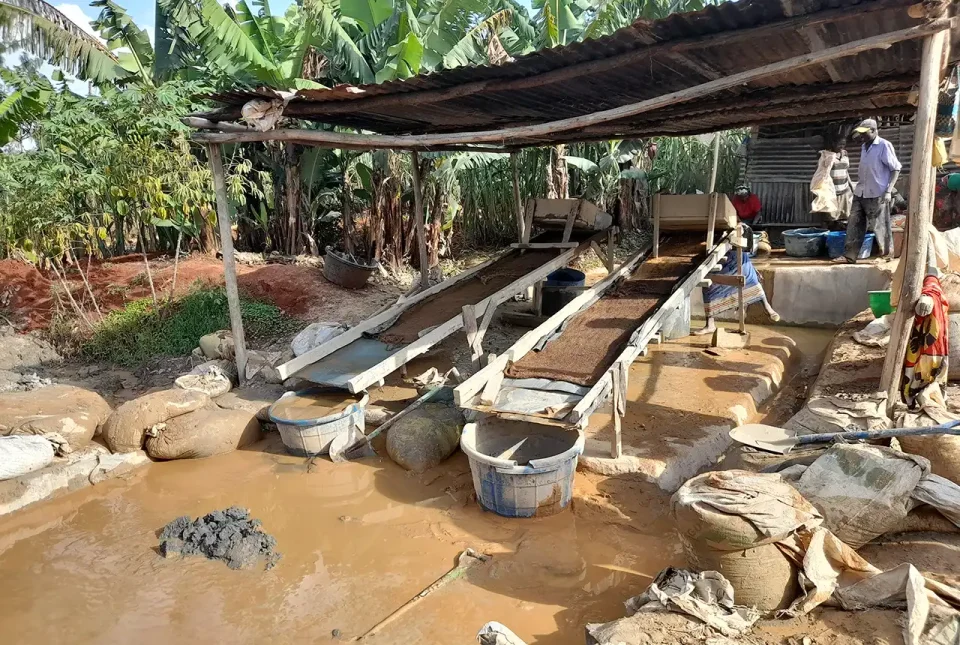
Linking geochemistry and health in artisanal and small-scale gold mining in the Kakamega-Vihiga gold belt, Kenya
09/01/2024
PhD candidate Maureene Auma Ondayo is investigating major and trace element exposure in the environment in Kenya, aiming to reduce exposure of humans to toxic chemicals.
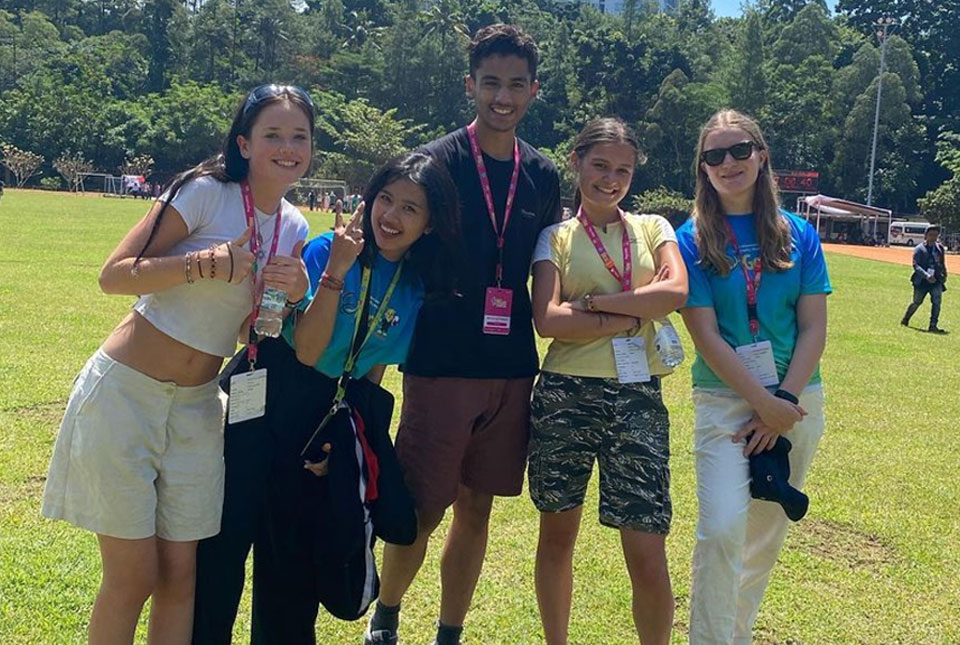
The Geography Olympiad: Bandung, Indonesia
22/12/2023
School student Dion Thompson joined Team UK at this year’s International Geology Olympiad in Indonesia. We hear from Dion’s mentor Anna Hicks, before Dion reports on the Olympiad itself.