In recent years, there has been a trend towards the collection of ever-more quantitative data, a movement that has so far been exemplified by the Tellus projects in Northern Ireland and south-west England. These projects consisted of series of surveys collecting both geochemical and geophysical data through combined ground and air surveys. The beauty and utility of these multidisciplinary surveys is uncovered not in the independent analysis of individual variables, of which there are many, but in the treatment of the large, multivariate datasets as a single system: a fully quantitative representation of the geo-environment as a whole.
Thanks to the current global explosion in big data analytics, we have more tools at our disposal than ever before to make sense of such complex data systems. We can use these tools — machine learning algorithms — to explore our data and to condense them into meaningful, evidence-based solutions for specific end users.
The maps reveals all the same features as a traditional geological map and more, all with transparent uncertainties and prediction accuracies in excess of traditional interpolation methods (cross-validated R2s up to 0.9).
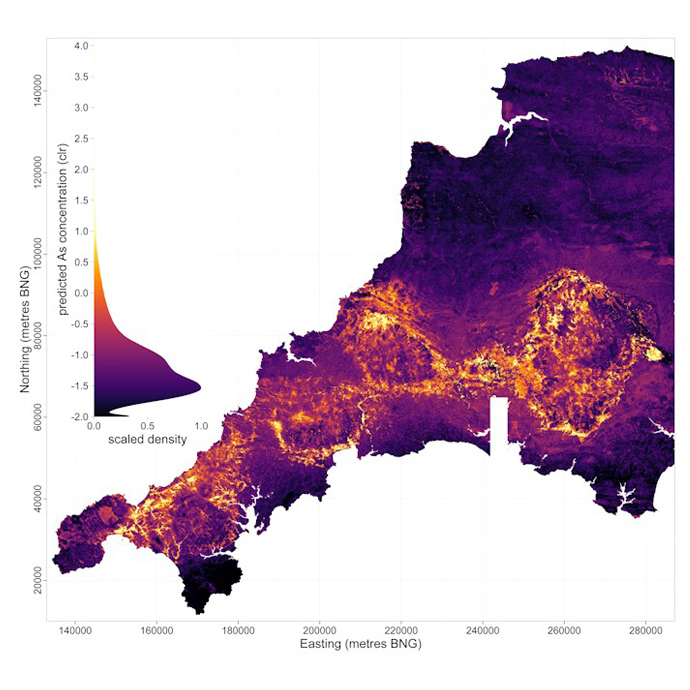
Arsenic enrichment in south-west England, produced using an artificial neural network in the MXNet framework. BGS © UKRI.
The use of machine learning has so far been focused on modelling and predicting geochemistry; after all, chemical composition is a direct quantification of what a rock or soil actually is and therefore provides an ideal data format through which to port traditional mapping workflows into the digital domain.
As interest grows in improving evidence-based understanding of the behaviour of the rocks beneath our feet, we will need to augment our data systems with measurements of a wider range of geo-properties. For example, in addition to data on chemical composition, we can incorporate data on mechanical properties, hydrological properties and so on in order to provide reliable decision-support tools for a wider user base.
For now, machine learning is successfully allowing us to translate multisensory, airborne-survey data into accurate, high-resolution maps of the chemical composition of the rocks and soils that support the foundations of our society.
Contact
If you want to discover more then please contact Russell Lawley.
You may also be interested in
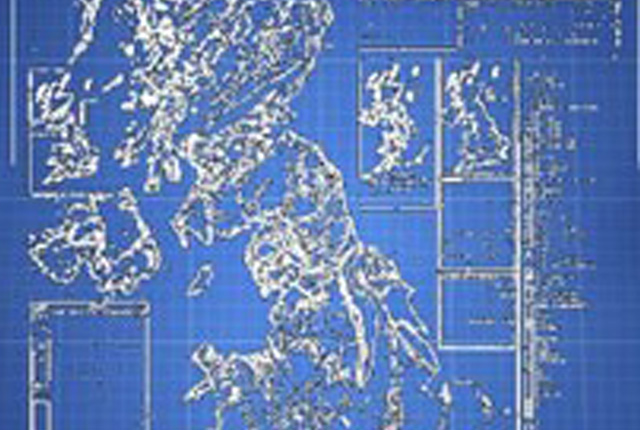
Product development
The properties and resources team publishes a broad range of geological data and information, including digital mapping, for all stakeholders.