Sensors are often used to observe the environment through the collection of data. Data analysis from multiple sensors may be used to support a decision, but the analysis may occur days or even years after the data was originally collected.
In some cases, sensors are used as switches to make automated real-time changes in a catchment with no human input: for example, opening a reservoir’s sluice gate when water in a reaches a critical level. Although these systems work well, their uses are limited.
For the last couple of decades, sensors have taken advantage of digital communication to connect with each other and telemeter data back to a local or centralised hub. More recently, cheaper telemetry and sensors, the push for open datasets, cloud storage and Earth observations have led to a vast increase in the volume, scale and availability of real-time environmental data.
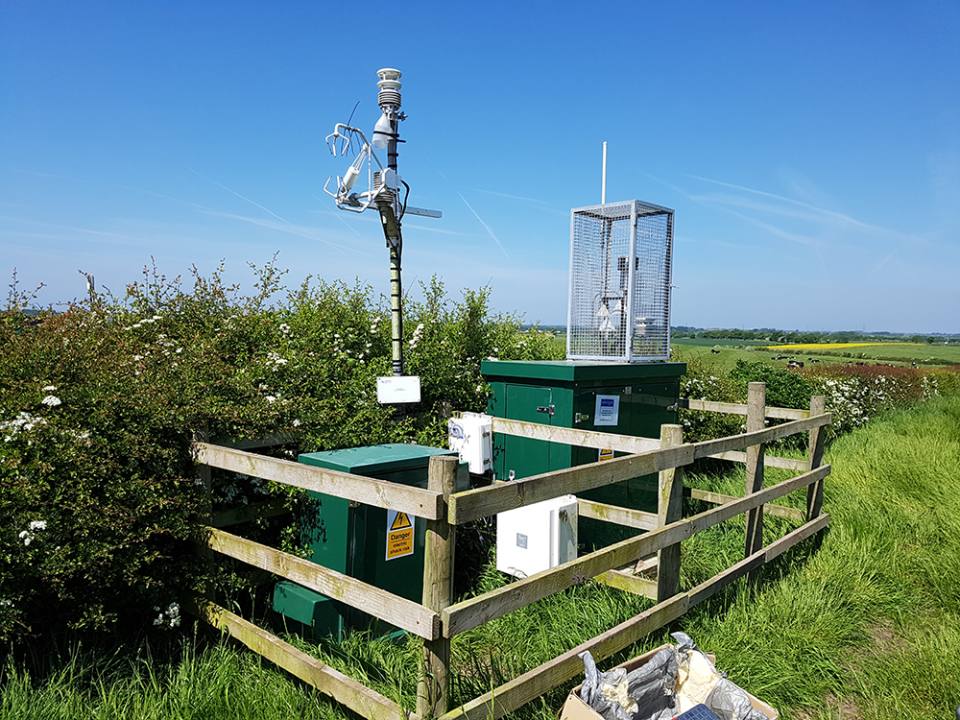
Eddy Covariance system installation. The EC system provides real-time information on soil–atmosphere gas exchange and can be useful for monitoring both natural cycles or anthropogenic releases of greenhouse gases from the ground. We are working with various stakeholders to assess the effectiveness of EC for real-time leak detection. BGS © UKRI.
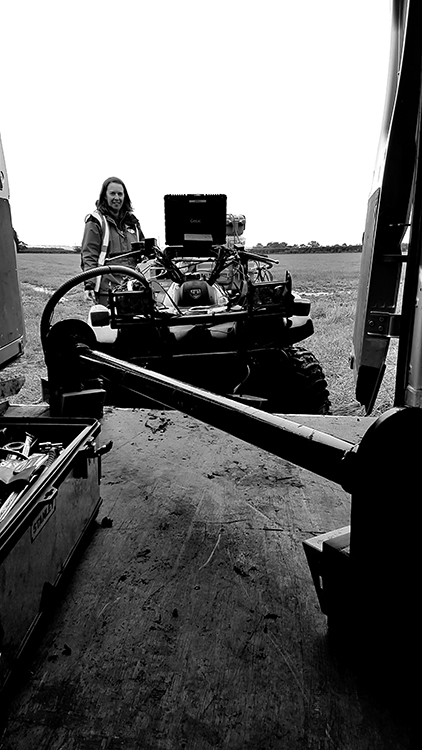
Setting up for mobile carbon dioxide (CO2) and methane (CH4) measurement. Mobile detection using an ATV allows rapid collection of near-surface gas concentrations over a wide area. These observations can be used to build a baseline understanding of gas release for a particular site or for the detection of natural or anthropogenic greenhouse gas hotspots on the ground. BGS © UKRI.
There is the potential for these datasets to revolutionise our understanding of the environment and reduce the time taken from observation in the field to delivery of analysis. The smart observation systems project will investigate how numerical modelling, machine learning and forecasting can use environmental data networks to support effective near-real-time decision making.
Digital twins
A digital twin is a numerical model of the physical environment synchronised to that environment through sensors. The continuous link between physical and digital allows near-time forecasting, experimentation and scenario analysis to be undertaken within a virtual model. The uptake of digital twins will enable stakeholders to optimise the impact of decisions before they are implemented.
Water and the dynamics of water in a catchment underpin all the benefits that ecosystems provide and play a huge role in shaping social, economic and environmental wellbeing. Under the smart observing systems (SOS) project, we are developing digital twins that represent the partitioning and flow of surface and subsurface water within catchments. Using real-time observation networks and weather forecasting to drive the digital twins, the SOS project will create flood, drought, soil moisture, groundwater level and storm surge forecasts across catchments at sub-daily to seasonal timescales.
Machine learning
Very short lead-time flood predictions (minutes to an hour) are considered an important research challenge, particularly in highly urbanised areas where timely warnings to residences can reduce flood impact. Process-based numerical models (including digital twins) are unsuited to this task due to simulation runtime.
Data-driven statistical models have a long tradition in flood prediction and are much quicker to run than their processed-based counterparts that, although efficient, are unsuitable for short-term prediction. The drawbacks of process-based and statistical models, combined with the expansion in data availability over recent years, have led to the use of machine learning (ML) techniques for flood forecasting.
Data-driven prediction models using ML are promising tools as they are quicker to develop and run, and can be used with minimal inputs. The SOS project will construct and evaluate ML prediction models for flood forecasting at differing scales and in different environments. In the future, the SOS project will combine these trained ML models with real-time data to develop automated, very short lead-time flood-alert systems.
Contact
If you want to discover more then please contact Andy Barkwith.
You may also be interested in
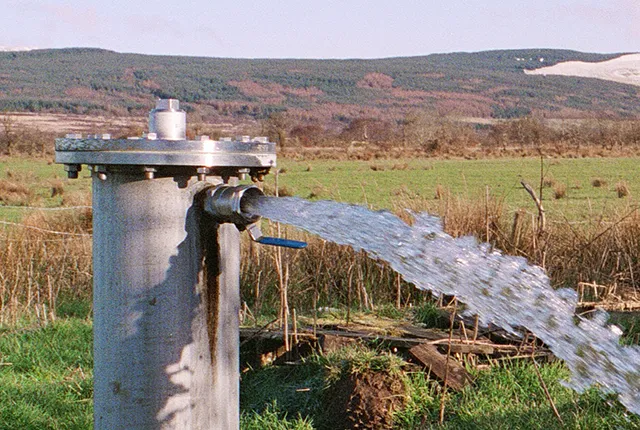
Groundwater research
Addressing issues related to the sustainability of water resources and quality, and the effects of environmental change on the water cycle, natural hazards, and human health.
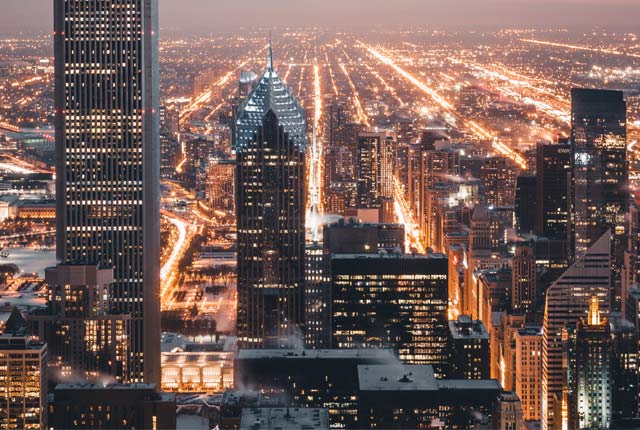
Urban geoscience
Providing solutions for urban land-use planning and sustainable development.